Demo
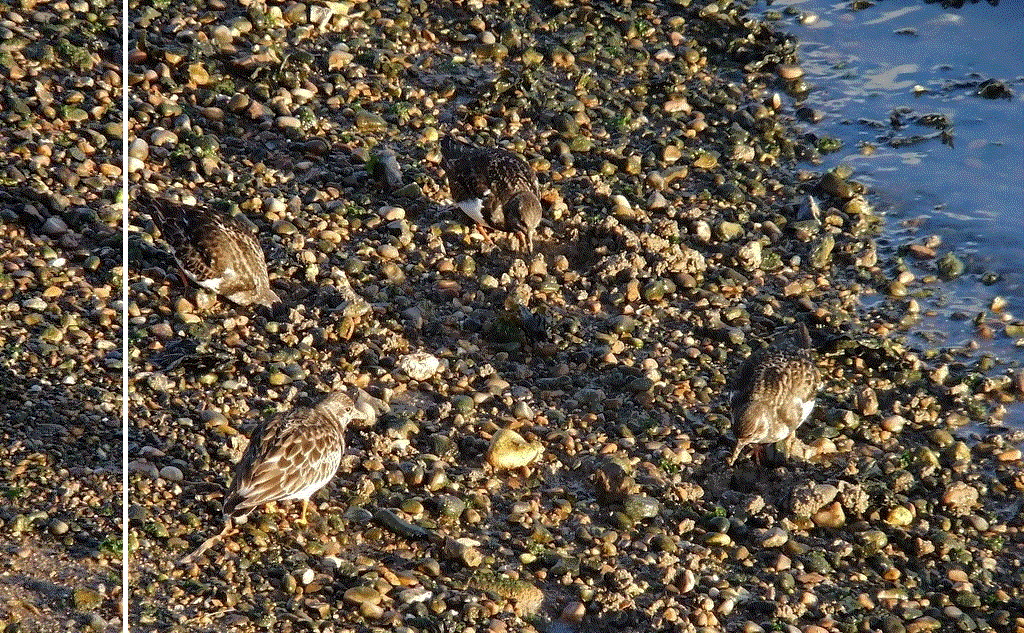
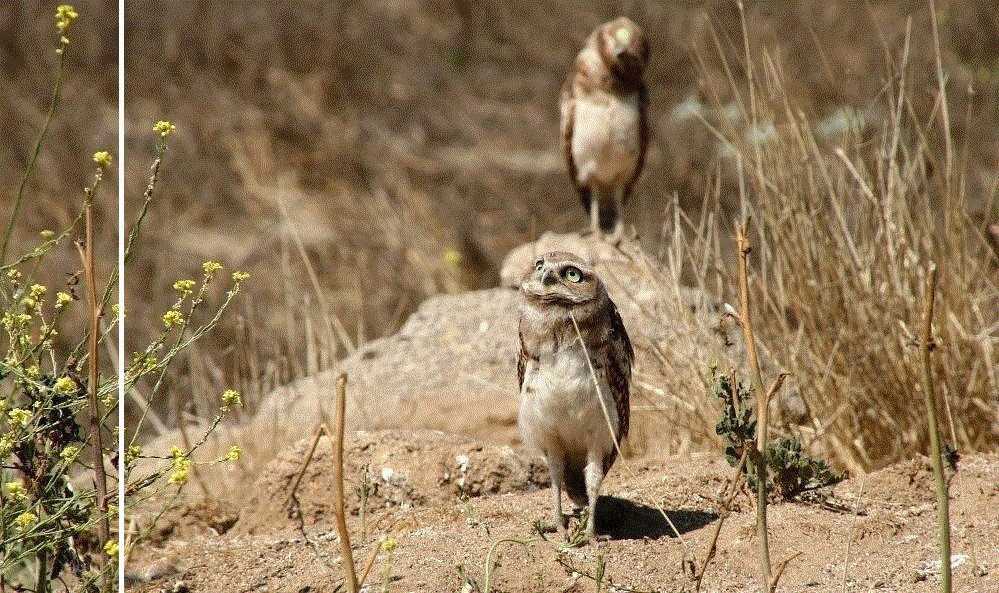
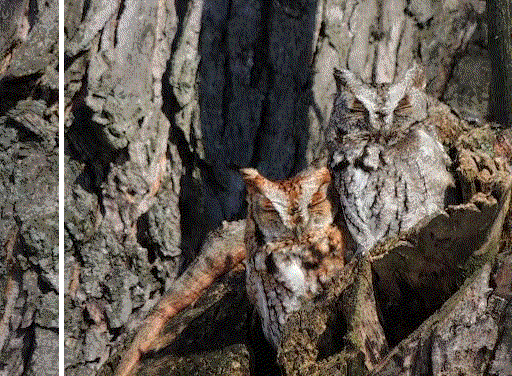
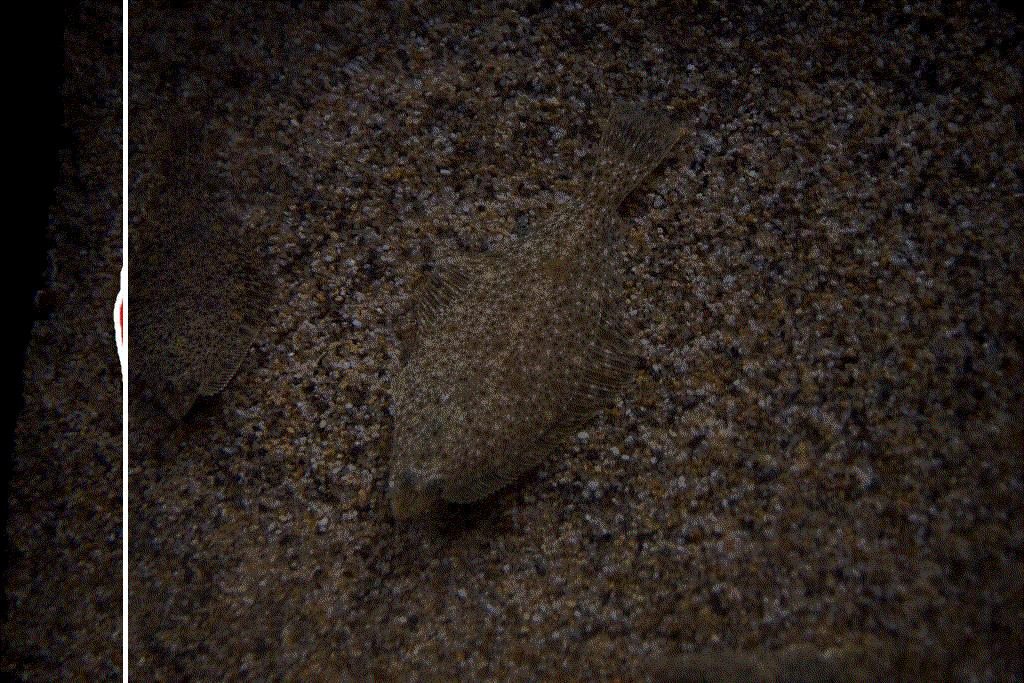
Overview

We present OSFormer, the first one-stage transformer framework for camouflaged instance segmentation (CIS). OSFormer is based on two key designs. First, we design a location-sensing transformer (LST) to obtain the location label and instance-aware parameters by introducing the location-guided queries and the blend-convolution feedforward network. Second, we develop a coarse-to-fine fusion (CFF) to merge diverse context information from the LST encoder and CNN backbone. Coupling these two components enables OSFormer to efficiently blend local features and long-range context dependencies for predicting camouflaged instances. Compared with two-stage frameworks, our OSFormer reaches 41% AP and achieves good convergence efficiency without requiring enormous training data, i.e., only 3,040 samples under 60 epochs.
Citation
@inproceedings{pei2022osformer,
title={OSFormer: One-Stage Camouflaged Instance Segmentation with Transformers},
author={Pei, Jialun and Cheng, Tianyang and Fan, Deng-Ping and Tang, He and Chen, Chuanbo and Van Gool, Luc},
booktitle={European conference on computer vision},
year={2022},
organization={Springer}
}
More Visual Results
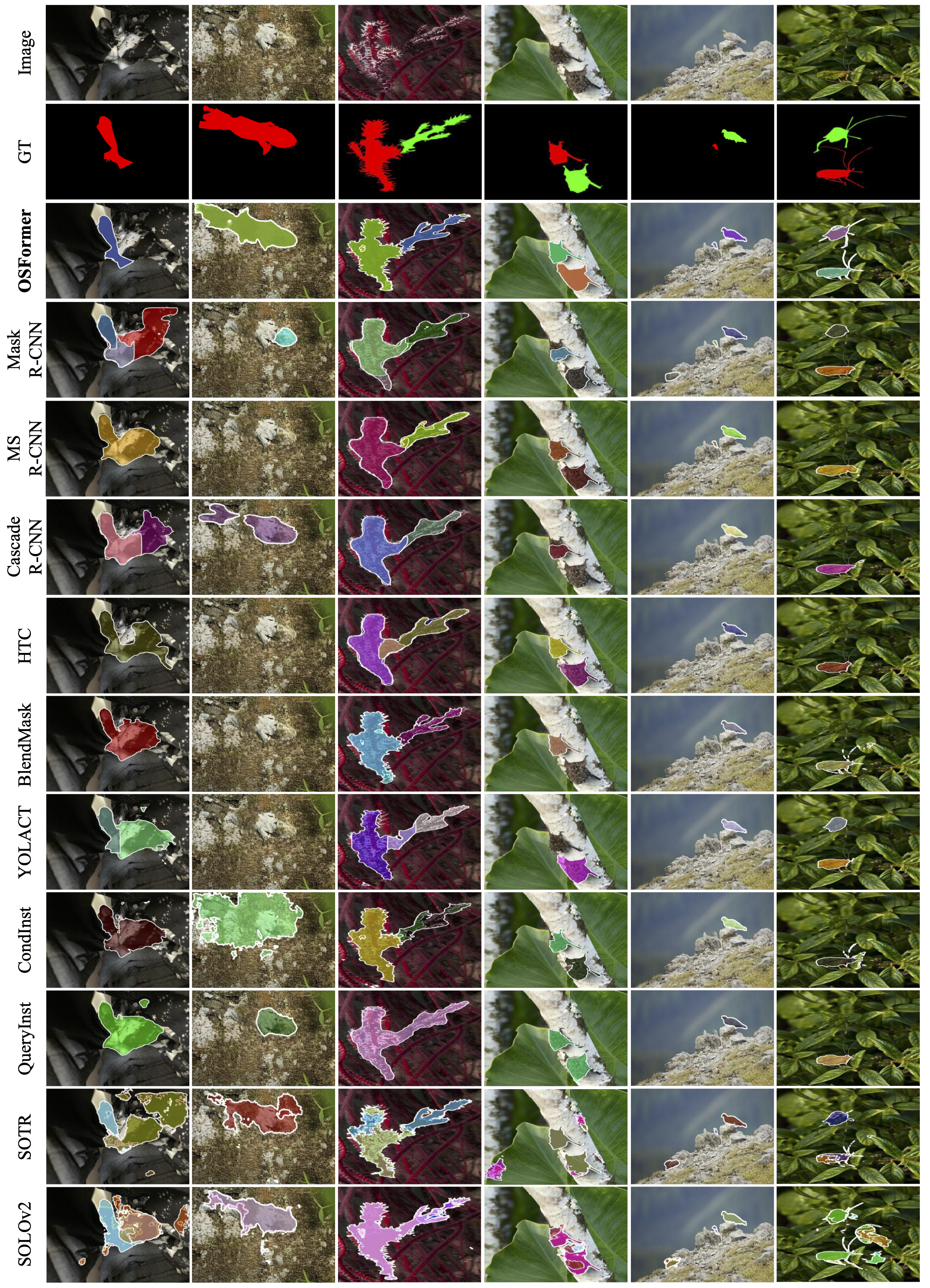
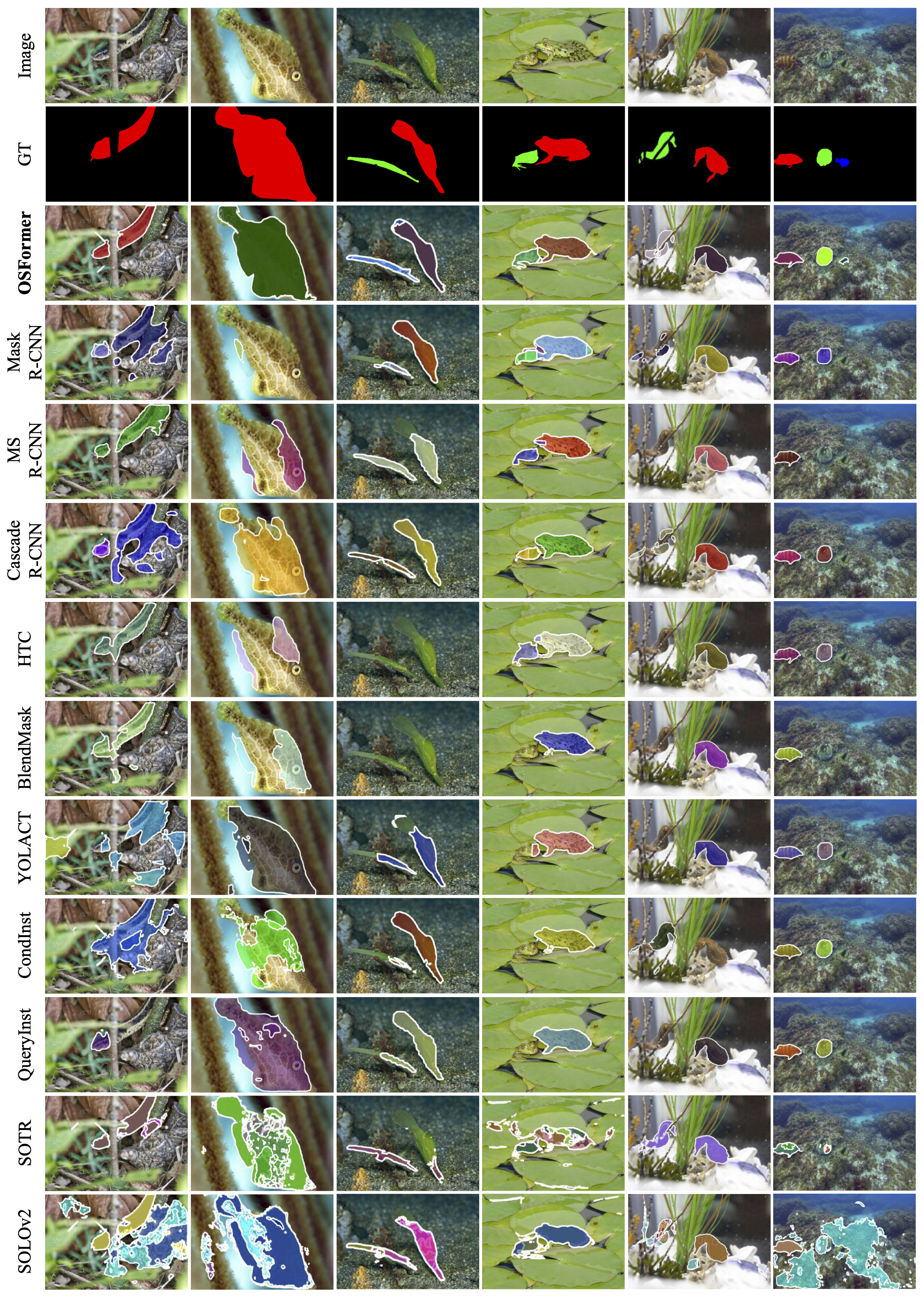